SNIPE: Sensor Network for Intelligent Predictive Enterprise. IoT / Cybersecurity and AI-based demonstrator for predictive maintenance in the foundry industry.
Name of demonstration
SNIPE: Sensor Network for Intelligent Predictive Enterprise. IoT / Cybersecurity and AI-based demonstrator for predictive maintenance in the foundry industry.
Main objective
The main objective of the demonstrator is to create a Smart Monitoring infrastructure for revamping 4.0 of non-digitalized Foundry process, predicting machine down-time collecting and analysing process data.
Short description
SNIPE project proposes an AI-based, modular decision support system for predictive maintenance and real-time monitoring of operation performance, to improve efficiency in the foundry and casting industry.
SNIPE demonstrator creates an end-to-end solution that goes from a sensor network, through a multi-protocol IoT Gateway, up to a Cloud Dashboard for data storage and analysis, with a dedicated cybersecurity infrastructure.
This demonstration has received funding from the European Union’s Horizon 2020 research and innovation programme under grant agreement No 825196.
Owner of the demonstrator
FAE TECHNOLOGY SPA
NACE
C26.1.2 - Manufacture of loaded electronic boards
Keywords
IoT - Cybersecurity - Artificial Intelligence - Predictive Maintenance – Revamping.
Benefits for the users
● Increase of productivity level (OEE performance index) and reduction of process instability that typically brings complex procedures to restore correct working conditions.
● Energy savings, in all conditions where energy consumption cannot be adjusted during process interruption and machine down-time (e.g. melting furnaces).
● Reduction of machine down-time through the application of AI algorithms and predictive maintenance technologies.
● Reduction of Cybercrime and hacking protection when the plant is digitally connected, through the application of best-in-class cybersecurity techniques.
● Easy transfer of information from the Smart Monitoring Cloud platform to the workers, with the use of a wearable device.
Innovation
New and modern 4.0 machines generally have sophisticated and integrated monitoring solutions, so as to prevent failure. However, most of the equipment that is currently in use in the heavy industry is not digital yet, and the business using it usually has to realize the maximum possible value, before replacing it. Consequently, maintenance is an issue, because the cost of machine down-time in heavy industry is high. Over the years, businesses have overhauled maintenance processes to alleviate down-time and improve effectiveness, and the scheduled maintenance has been mostly implemented with pros and cons.
Within this scenario, SNIPE demonstrator proposes the use of prognostic and health management AI algorithms, to control the production process and plan maintenance only when really necessary. Moreover, the introduction of an IoT modular infrastructure – like the one proposed in SNIPE demonstrator – lets companies monitor critical processes with low investment w.r.t. buy new equipment, and open the way to prevent failure through AI based elaboration of data.
Risks and limitations
Technical limitations are mainly related to the implementation of electronic and IOT sensors in the industrial environment: a detailed system architecture planning phase must be considered so to anticipate possible interferences / problems in the wireless data transmission. Risks can emerge when the production plant is web-connected, but the application of Cybersecurity Hardware and Software technologies can protect data and IT infrastructures from possible external attacks and data leaks.
Technology readiness level
6 - Safety approved sensors and systems are commercially available
Sectors of application
Foundry and casting industry (heavy industry): predicting maintenance and real-time anomaly detection to reduce energy waste and improve efficiency., | Manufacturing companies: revamping production processes with the application of IoT Sensors to collect critical data from the field (e.g. engine vibration, current consumption...)., | Manufacturing companies: machine-human interface through the use of dedicated wearable IoT devices to transfer information on process and cycle performances. .
Potential sectors of application
Plastic Moulding companies: smart tracking of production goods along the production process with application of tracking sensors. | Logistic companies: predictive maintenance application on belt conveyor transfer process. | Airport: predictive maintenance of the belt conveyors to transfer baggage in the airport.
Hardware / Software
Hardware:
IoT sensor node: based on STWIN.BOX device Wireless Industrial Node, collecting data (vibration, temperature, humidity and energy consumption) from belt conveyor engine and transmitting via Wi-Fi to Gateway.
IoT Gateway FAE Technology KITRA GTI, Linux Ubuntu machine with Wi-Fi, 4G LTE / GPS, Ethernet connection based on Samsung CPU (https://fae.technology/en/prodotti/kitra-gti/ ).
Secure Element for IoT sensor node based on NXP SE050 security crypto component.
Wearable device based on STM32 MCU, Espressif ESP-WROOM-32 Wi-Fi module, vibromotor and LED
Secure Element USB plug for IoT Gateway based on NXP SE050 security crypto component.
Software:
Cloud Dashboard, data storage and data analytics based on MangroviaIoT platform (https://www.mangroviaiot.com/ ).
Photos
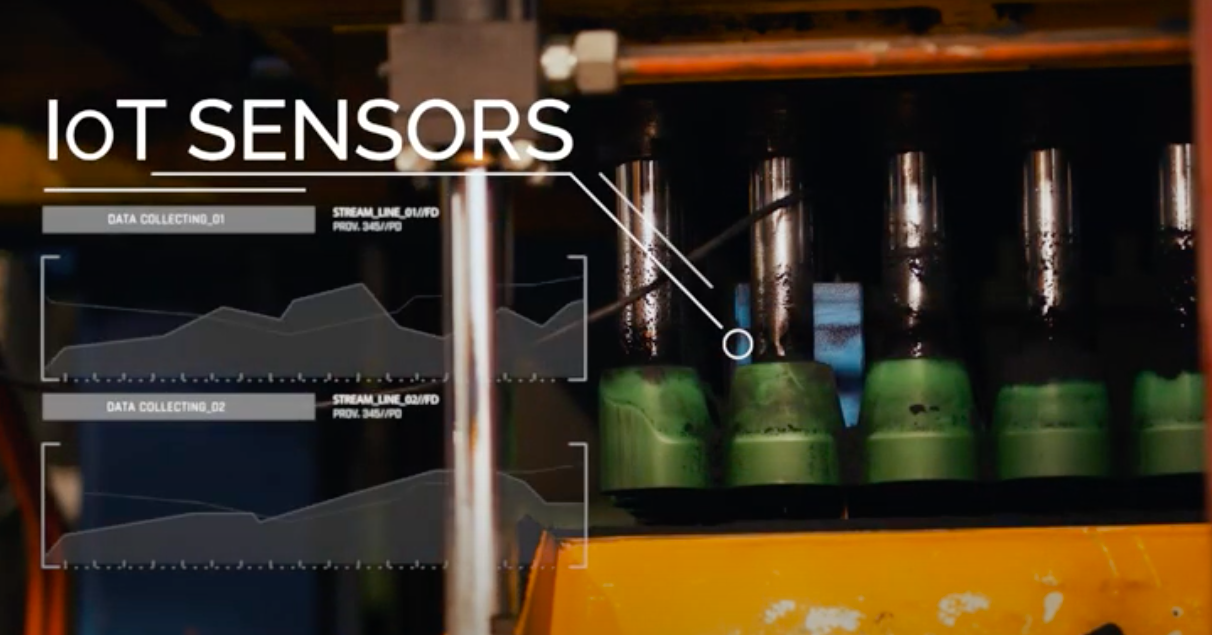
No modules assigned
Trainings
To learn more about the solution, click on the link below to access the training on the Moodle platform
Sensor Network for Intelligent Predictive Enterprise